Sustainable Internet of Healthcare Things for Smart Cities
Written by Kuldeep Singh Jadon, Nitin Gupta, and Lalit Awasthi
In the development of smart cities, smart healthcare stands out as a crucial sector, playing a significant role in shaping these advanced urban centers. A key aspect of smart healthcare is the emerging Internet of Healthcare Things (IoHT), which has demonstrated particular importance in the wake of the COVID-19 pandemic. However, building a sustainable IoHT environment comes with several major challenges. These challenges include ensuring energy efficiency, safeguarding data security and privacy, addressing interoperability issues, achieving scalability, overcoming network challenges, and adhering to relevant standards and regulatory policies. Among these challenges, ensuring energy efficiency is of utmost importance in achieving a sustainable IoHT environment. This article discusses important factors and considerations in the improvement of energy efficiency in IoHT environments, which play a crucial part in the development of sustainable smart cities.
Introduction
Sustainability is dedicated to discovering cost-effective methods for mitigating environmental impact while maximizing the utilization of computing infrastructure and associated tools [1]. The principal objective of sustainability is to create, design, produce, utilize, and dispose of devices in a manner that supports a smart global ecosystem [1]. Green computing, within the context of sustainability, places primary emphasis on efficiently reducing energy consumption, greenhouse gas emissions, hazardous waste disposal, and recycling, as well as non-biodegradable industrial waste. There are various challenges in achieving sustainability in IoHT. The most important and pressing of these challenges are summarized in Figure 1. Among these challenges, energy efficiency is most important for achieving sustainability.
Figure 1: Challenges of Sustainable IoHT.
Factors for Improving Energy Efficiency in IoHT
Figure 2: Important factor used to improve Energy Efficiency in IoHT.
As shown in Figure 2, three major components affect energy efficiency in IoHT; hardware design of different devices, data communication and computation management, and software optimization.
Hardware Design: In hardware design, there are a number of factors that impact energy efficiency which depend on whether the device or sensor is implanted inside the body or on the body. When a device is implanted on the body, an external power source is available. Therefore, the accuracy of the output produced by the device or sensor can be of sole focus. However, if the device or sensor is implanted in the body, the roles of the sensor, actuator, and microcontroller become crucial, since the device must be powered by an internal source or battery. To manage battery life of the device, sleep cycle management is essential. The previous history of the sensor can also be used to obtain information about sleep cycle [2].
Another approach that aids in reducing battery power is collaborative sensing. Collaborative sensing allows sensors to share data and resources, which can lead to reduced energy consumption [3]. Collaborative sensing can improve the accuracy of sensing by combining data from multiple sensors, since combined data is more likely to be accurate than the data from a single sensor. Collaborative sensing can increase the scalability of sensing by allowing sensors to form clusters. This allows sensors to share data and resources with other sensors in the cluster, which can improve the overall efficiency of the system [4].
Communication and Computation: Another approach to enhance energy efficiency involves focusing on communication and computation. Various organizations like IEEE are dedicated to creating standards and protocols enabling communication and computations for IoHT. The implementation of these standards can contribute significantly to creating a sustainable environment. Various application protocols such as Data Distribution Service (DDS), Constrained Application Protocol (CoAP), Advanced Message Queuing Protocol (AMQP), Message Queuing Telemetry Transport (MQTT), MQTT for Sensor Networks (MQTT-SN), Extensible Messaging and Presence Protocol (XMPP), Hypertext Transfer Protocol (HTTP), Representational State Transfer (REST) and some infrastructure-based protocols such as RPL, 6LoWPAN, and IEEE802.15.4 play a crucial role in enabling IoT communication [5].
While these protocols are efficient, there is a growing demand for lightweight protocols to cater to devices that require minimal power consumption while maintaining optimal efficiency. A few lightweight protocols currently being employed to address this need are summarized in Table I.
S.No | Protocol | Author | Key Points | Low Power Consumption |
1 |
LWM2M | [6] | An Open Mobile Alliance (OMA) client-server protocol, it is founded on IETF protocol and security standards. Connectivity and resource monitoring, as well as firmware updates, are just a few of the functions it offers. | Yes |
2 | CoMI | [7] | Accessing the resources of Internet of Things devices that have been described in YANG or SMIv2 translated to YANG via the CoAP protocol is made possible by this network management protocol. | Yes |
3 | 6LowPAN-SNMP | [8], [9] | IPv6 Low-Power Wireless Personal Area Network(6LoWPAN) with Simple Network Management Protocol (SNMP) is called as 6LowPAN-SNMP. It’s optimised for use in networks with limited resources, and it provides access to SNMP functions over IPv6 Low-Power Wireless LANs. To do this, the quantity of SNMP messages is reduced via a compression process applied to the SNMP header. | Yes |
4 | NETCONFlight | [10] | The Internet Engineering Task Force (IETF) announced NETCONF Lite [25], a lightweight network management protocol designed for resource limited IoT and some other applications.When it comes to controlling and monitoring network components that are separated into the control and data planes, NETCONF is in line with SDN principles. | Yes |
Software Optimization: The last important factor is software optimization. This can be achieved using different strategies, such as Artificial Intelligence (AI), Machine Learning (ML), Deep Learning (DL) and edge & fog computing. AI and ML algorithms can be used to make intelligent decisions about task offloading on edge and fog. These algorithms can analyze various factors, such as task requirements, available resources, network conditions, and energy profiles to determine optimal offloading strategies.
By considering these factors, AI and ML can minimize energy consumption by efficiently distributing computational tasks between local and remote devices. This is done by taking into account the trade-off between energy consumption for local execution and data transfer overhead. The goal is to achieve energy-efficient task execution while balancing the impact of data transfer on energy consumption and latency [11] [12].
Additionally, fog and edge computing play crucial roles in addressing various challenges in the context of the IoHT environment. Firstly, these technologies effectively reduce communication delays by processing data in close proximity to its source, thereby minimizing network latency. This characteristic proves particularly beneficial for applications that rely on real-time data processing, such as transferring CT-scan data, riding self-driving vehicles, and utilizing industrial automation systems [13]. Secondly, fog and edge computing enable the distribution of workloads across multiple devices and gateways instead of relying solely on a centralized cloud architecture. This distributed approach facilitates easier scalability of IoT systems, reducing the burden on the cloud and enhancing overall system performance, which results in improved efficiency and resource utilization [13]. Thirdly, the local processing of data and reduced reliance on transporting data to the cloud contribute to cost reduction, making the IoHT environment more financially viable and cost-effective [13]. Lastly, fog and edge nodes can operate in offline mode, even when the cloud node is inaccessible. This capability ensures continuous functioning and resilience of the IoHT system [13]. Therefore, the integration of fog and edge computing in the IoHT system enhances overall efficiency and sustainability by reducing communication delays, improving scalability, optimizing resource utilization, lowering costs, and ensuring reliable operation, even in challenging network conditions.
Conclusion
By improving hardware design, communication and computation efficiency, and software optimization, it is possible to attain energy-efficient IoHT devices for various health and elder care applications in a smart city. This is crucial for establishing sustainable IoHT ecosystems that can operate for extended duration without the need for frequent battery replacements or recharging, making it particularly suited for elderly care applications. A sustainable IoHT can play an important role in the establishment of future smart cities.
References
- S. K. Das and H. K. Palo, “Internet of things (iot) application in green computing: an overview,” Advances in Greener Energy Technologies, pp. 85–102, 2020.
- N. Kaur and S. K. Sood, “An energy-efficient architecture for the internet of things (iot),” IEEE Systems Journal, vol. 11, no. 2, pp. 796–805, 2015.
- S. K. Dhurandher, I. Woungang, N. Gupta, R. Jain, D. Singhal, J. Agarwal, and M. S. Obaidat, “Optimal secondary users selection for cooperative spectrum sensing in cognitive radio networks,” in 2018 IEEE Globecom Workshops (GC Wkshps). IEEE, 2018, pp. 1–6.
- X. Deng, L. T. Yang, L. Yi, M. Wang, and Z. Zhu, “Detecting confident information coverage holes in industrial internet of things: An energy-efficient perspective,” IEEE Communications Magazine, vol. 56, no. 9, pp. 68–73, 2018.
- A. Al-Fuqaha, M. Guizani, M. Mohammadi, M. Aledhari, and M. Ayyash, “Internet of things: A survey on enabling technologies, protocols, and applications,” IEEE communications surveys & tutorials, vol. 17, no. 4, pp. 2347–2376, 2015.
- S. Rao, D. Chendanda, C. Deshpande, and V. Lakkundi, “Implementing lwm2m in constrained iot devices,” in 2015 IEEE Conference on Wireless Sensors (ICWiSe). IEEE, 2015, pp. 52–57.
- M. Veillette, P. der Stok, A. Pelov, A. Bierman, and I. Petrov, “Mar. 2020. coap management interface,” Internet-Draft draft-ietf-core-comi-09, Internet Engineering Task Force . . . , Tech. Rep.
- Y. F. Do Rosario and X. Fafoutis, “Comp: Efficient monitoring for constrained embedded devices,” in 2020 IEEE Symposium on computers and communications (ISCC). IEEE, 2020, pp. 1–7.
- H. Choi, N. Kim, and H. Cha, “6lowpan-snmp: Simple network management protocol for 6lowpan,” in 2009 11th IEEEinternational conference on high performance computing and communications. IEEE, 2009, pp. 305–313.
- A. Mavromatis, C. Colman-Meixner, A. P. Silva, X. Vasilakos, R. Nejabati, and D. Simeonidou, “A software-defined iot device management framework for edge and cloud computing,” IEEE Internet of Things Journal, vol. 7, no. 3, pp. 1718–1735, 2019.
- Y.-L. Jiang, Y.-S. Chen, S.-W. Yang, and C.-H. Wu, “Energy-efficient task offloading for time-sensitive applications in fog computing,” IEEE Systems Journal, vol. 13, no. 3, pp. 2930–2941, 2018.
- N. Charef, A. B. Mnaouer, M. Aloqaily, O. Bouachir, and M. Guizani, “Artificial intelligence implication on energy sustainability in internet of things: A survey,” Information Processing & Management, vol. 60, no. 2, p. 103212, 2023.
- S. Das and P. Guria, “Adaptation of machine learning in fog computing: An analytical approach,” in 2022 International Conference for Advancement in Technology (ICONAT). IEEE, 2022, pp. 1–11.
This article was edited by Bernard Fong.
To view all articles in this issue, please go to August 2023 eNewsletter. For a downloadable copy, please visit the IEEE Smart Cities Resource Center.


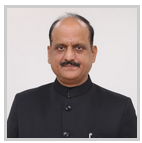
To have the eNewsletter delivered monthly to your inbox, join the IEEE Smart Cities Community.
Past Issues
To view archived articles, and issues, which deliver rich insight into the forces shaping the future of the smart cities. Older eNewsletter can be found here. To download full issues, visit the publications section of the IEEE Smart Cities Resource Center.